Introduction
Thousands of new malicious apps are being released for mobile devices every day. And thousands more variations of older malware are being released too. Unfortunately, many of these new/old threats are not being detected by the existing mobile malware technology. Organizations need next generation machine learning-based solutions that can effectively detect these unknown malware variants without updates and protect their devices and users.
Zimperium is the only mobile security solution delivering on-device, machine learning-based detection of malicious apps and has become the benchmark standard for machine learning-based detection of mobile malware.
Yes, that is a bold statement… but we stand ready to prove it.
What is the single best way to prove the effectiveness of a machine learning-based mobile anti-malware engine? Detecting mobile malware variants that have never been seen before.
This post documents a series of malicious apps that were detected by z9… samples (including ones of well known families that have been around for years, such as Anubis and BankBot) that were publicly unknown. We conducted a webinar to provide additional context, which can now be viewed on-demand.
Frequently, security researchers and vendors focus on the latest malware family that hit the news while forgetting about older campaigns that continue to pose a real risk to the end user. In this post, we will highlight recycled and evolving malware families bypassing existing mobile anti-malware technology (ie. signatures, cloud-based analysis) to reinforce the importance of having an advanced malware detection engine, such as z9.
Malware family correlation and identification
The strength of Zimperium’s z9 for malware engine is its ability to detect unknown malware using its on-device machine-learning classifiers. As unknown detections are triggered, the samples and their metadata are thoroughly analyzed in our systems. While performing our analysis on some of the unknown samples we noticed a strong correlation between the new, unknown samples and previously known, well-covered malware samples. This prompted us to dig deeper.
We extracted a subset of samples that were not known to the industry or resident in public malware repositories. The samples were then run through our z9 for malware family classification engine and grouped into SMS Fraud, Banker, Dropper,Riskware and Locker families. More precisely, we linked the unknown samples to known samples that are part of the following known malware campaigns:
- SMS Fraud (Avito)
- Banker (Anubis/Bankbot older non-obfuscated versions)
- Banker (Anubis/Bankbot updated obfuscated versions)
- Dropper/Banker (HQWar trojan dropping Bankbot payloads)
- Riskware/Dropper (Hidden Adware, Triada and spyware payloads)
- Locker/Ransomware (Chinese lockscreen locker)
In the following section, we will briefly analyze the attack vectors and behaviors found in the malware samples.
Involved Actors
Far from being simple malvertising families, the analyzed campaigns pose a real and serious security risk to users:
SMS fraud – Avito:
E-commerce apps are also a profitable target for malware authors. During this research, we found several samples targeting clients of the Avito market app, a Russian classified advertising platform with presence in several countries such as Morocco and Egypt. In the past, malware authors have used the information posted in this online store to distribute malware via SMS [1].
The campaign we found seems to be closely related with this group of old samples as they share parts of its implementation, such as the string decryption routines or the network communication logic. Among the malicious capabilities of the fake Avito family, it is possible to find SMS stealing techniques, installation of rogue device administration policies or device information exfiltration. Despite the fact that many blog posts discussing this family date from 2016-2017, our findings showed that an early and much simpler strain of fake Avito samples is still in the wild. During analysis, some clear differences came up:
- Device administration capabilities: While the main entry point of recent samples of the fake Avito family leads to the installation of a device administration privilege, the sample we analyzed requires manually triggering one of the services exposed in the manifest to display its malicious behavior. Although it also contains the logic to install a device administrator, its policy group is empty.
- Network traffic: The found samples generated far less traffic during analysis than the reported examples. During our research, we discovered how it retrieved a JSON document containing templates of what seems to be 2FA SMS. Such file is retrieved from 213.166.68.24 which, according to VirusTotal, was used to host several domains whose URL have been found in other Avito samples.
z9 detection results:
2e2cac6f6e55d1eab273b73cf5b3b787d26b26eff101b6ee2fd9ab2ebb470d91: Confidence: 100.00, Family: malware
0b4b9b25180b18f973d58b49bd6c8a2ef94d19ab0a884e6dfcac525b161e0c14: Confidence: 100.00, Family: malware
Some of the related samples are:
9909d3fc626bfe2c715a689d3a3e81a63ee5b892b2072042a53ed368d85e9cb1
3427862a88b3feb9a64bbfff087e519bcd9eca87f04fb4f70f715b12080c71b5
5e20f0f456bb91409303c822aedffaf1a813ea9bee86a88e58bd63a60149eafb
5b98ba917919a4acd91b082df9bb6d5e4d2f9d53aa9e126c14ee68f559240d60
Ransomware / Lockers:
In the last few years, ransomware and lockers (apps that encrypt data on the device) have rapidly become one of the most prevalent and harmful malware threats for both mobile and desktop devices. Malware authors take advantage of inexperienced users, luring them to installing malicious programs that block/brick the device or encrypt all its contents, asking for a ransom before allegedly taking the device to usable state.
During our research, some of the app lockers stood out. After a brief inspection, we found no special efforts were made to hinder analysis (i.e no obfuscation or anti-analysis techniques). In fact, we noticed the samples were some very basic versions of the old “SLocker” malware family, initially reported by TrendMicro some years ago [2] but yet unknown to online analysis platforms and AV vendors. As is common in Android lockers, the sample abuses the device administration API to gain control over the locking screen of the device, forcing the user to pay a fee to recover the access to the device.
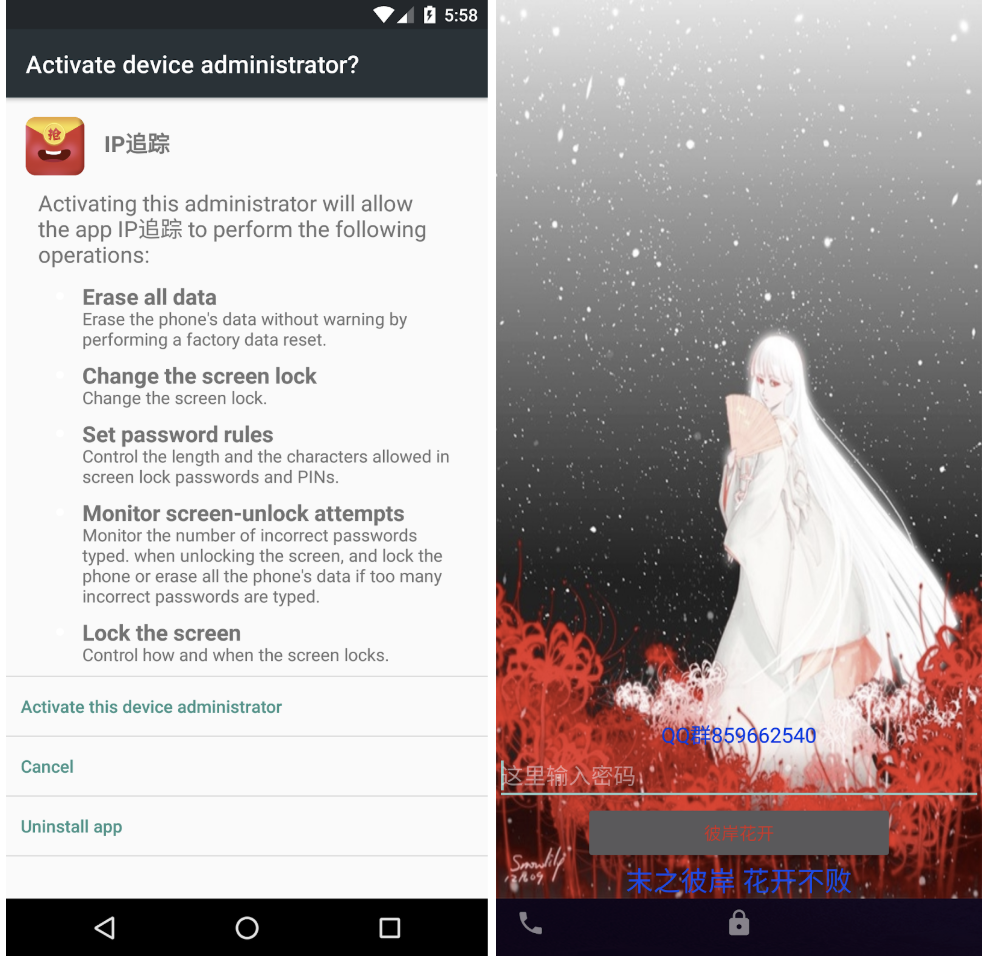
As depicted in the above images, the device is completely locked once the device administration policies have been activated and solely displays an EditText field with the hint 这里输入密码 (Enter your password). From a technical point of view, the sample does not feature any sophisticated or advanced characteristics. In fact, the algorithm employed to locking the screen is quite easy to reverse and eventually bypass. The authors employed two different techniques to lock the user away from the device: manipulating the layout settings and changing the unlocking password using the gained device administration capabilities. Both locks are easy to bypass as they both rely on the same logic. Roughly, the method that checks the user input takes the contents of the correspondent files (gdmm.txt in the case of the layout lock and pin.txt in the case of the device Administration lock), using its content as a parameter for the function depicted in the following snippet:
As can be seen, most of the content of the function can be ignored. It just performs replacements and just after, it undoes such substitutions. At the end of the day, the logic can be stripped to:
- Decode from Base64 the parameter string.
- Reverse it and decode from Base64 again.
Following these steps, both unlocking codes can be easily retrieved: 000316 & qqqq. It also seems this sample belonged to an early batch of SLocker samples.
z9 detection results:
113efd6c1956eb9e1f94ea04ff6ef63aaca912e3930acf8346d3696fd3736f23: Confidence: 100.00, Family: trojan
e6be2b3c3e89a314c4247ebfbf56b511ac38aba4acda7e300cdc50e6342c465d: Confidence: 100.00, Family: trojan
Some of the related samples are:
7d614c22c6be78cc5cff276a3da11894540f48a3d67c9a9e48dd34bc9753a064
04a64dd0da3070185ecae613f6af92ffb1c8c3e4901250859cd4d8b71722a699
a0866876adb4fdc5c36a1a430340f8067555b27ee4079f1ec6c4b56d8ebf5857
734ac8e659cb96fa450a24ae482d7692e2766312d4da53fbcb7bd27d23019bfc
Bankers:
The analyzed banker samples are part of (or derived from) Anubis, Bankbot and HQWar malware families. In particular, some samples were dropping very old versions of the first Bankbot payload. Generically speaking, all of them have been repurposed on several occasions during the years and tailored to different tasks.
Anubis has been spotted in cyberespionage, ransomware and banker scam campaigns, every time featuring updated or improved evasion mechanisms (e.g. at the start of 2019 it used a motion-sensor based anti-emulation technique). The main malware features are:
- Disable Google Play Protect
- Complete access to the SMS
- Take screenshots, record audio or lock the screen
- Monitor applications and open overlays with custom URLs
- Complete access to the Device Administrator configurations
- Complete access to the files with the possibility to encrypt them
- Using Twitter and Telegram as covert communication channels
- Steal device’s information like contacts, unique identifiers, processes list, GPS location
HQWar has been active since early 2016, had a peak in 2018 and is still alive in 2019, acting as an infection vector for many malicious campaigns. It implements a wrapper that decrypts and dynamically loads an embedded payload. The malicious actor relying on HQWar can sign the sample with different certificates: randomized, Google or private one.
Bankbot is one of the most famous banking trojans that has plagued Android users since 2016. During the years, it evolved to add an ever-growing list of targeted bank (and other) applications and greatly improved the string and class obfuscation used by the main payload. The principal attack vector is abusing Device Administrator and Accessibility services to trick the user into giving additional permissions. Once a bank application is launched by the user, the malware spawns an overlay on top of the legit activity mimicking the official app’s user interface. Once the victim inserts their credentials, they are sent to the C&C and the malware usually shows an error message saying that the bank services are not currently available.

z9 detection results:
26ace4765f2837a67c9158aa840698338593a35f217b5ea1f617153d4c98179f: Confidence: 100.00, Family: banker
ca0dad8c0d7daecd12de79f6ebabc33979e4c3dee688418b56efc01fe9b65bfc: Confidence: 100.00, Family: banker/malware
8322d5c6766fe87375afb32f48539bdf234c1adaacf889434dbaf059e2823095: Confidence: 99.96, Family: banker
Some of the related samples are:
d20e9a10e37a310904d70a1b68cafc1985767d9ec026142e7e5f33ade56dcc03
51648004f7c83d00c5deba460838be2b0b2eb33cdb9bb133a2ff36003f43e687
e9e35896811349f867c961118a409ff750918955daf76b37989b0aa89da8598f
6cf4b52430005a0af670630fbc927db2736f4827ae7352fa30466cfef1b84f30
53b6cba10003af1392b9f30c98699b891711ffb94be2812d184a40abfd890641
88eb93c07cfbf33ae0535f4500c43fa0ec58375ba7b6329283c71e47ecc555b3
4730a8937b6b75cc1bdfe86889aebc1f3e6823147fd5d077c3a8b488c6473a7d
2d8c7e26be34608a9a78a7b9d0f8252f1ee7a910fbb540521f49af0c6ad513a8
37c72cdf6d2eee61fa5dd5bee761e8eb5976207bff7ee8877cf8f3c2a1c32200
Riskware:
A sample should be considered “riskware” when it poses risks that may be unacceptable in certain environments. In the filtered set of samples, we found applications loading aggressive or hidden advertisements, collected unique information about the device and loaded paid subscription URLs without the user knowing about it.
It’s not uncommon to find legit applications embedding a lot of third-party advertisement SDKs that at some point turn malicious. Many advertisement SDKs turned malicious without changing their code. One of the most common attack vectors consists of loading a Javascript script downloaded from an external server and executing it inside a hidden WebView to control the actions on the loaded page (e.g. faking the clicks on ads or paid subscriptions). Based on some conditions (e.g. GPS location, usage of the device, time), the malicious behaviors may or may not show.
z9 detection results:
b93fae996fc9d6ded5f201627ed09c736a98eba7db2dfb53c65b33f7bb759e63: Confidence: 91.22, Family: riskware
Related hashes:
38f66740ac8221d4b41e204b89452ffc65675717ab9a7b54e6b1a888cbcd8e22
0b6f14760a1e72de7aef4a9e7091dbd84d4122b4bcf3d890a9c7fa6e2d26c9ac
31c7e93c30964add5fd9838f54dc53de16ed97f46aa13322be3bf77752695afc
c7f723019419a9c0a74a490658a41a13bb83c86e76052778a32aa43397b15113
Dropper:
Droppers are one of the most common families of malware targeting the Android platform and are very similar to the method that is found on desktop platforms.
The purpose of this kind of sample is to download and place a malicious payload in any folder inside the device storage space to be later loaded and executed. This allows the sample to leverage a secondary payload that may go undetected by security vendor detection methods. During our research, two samples were found that remain unknown to AV vendors according to VirusTotal data – despite vendors detecting earlier samples of the sample families. However, our correlation process and detection engine allowed us to link them to other known droppers already detected by our AI-based detection engine.
z9 detection results:
837541c220f0eb9e2e83e9aea81ad1a83b53a857c48646fc1e9905d1a6fe7d45: Confidence: 100.00, Family: trojan
9d05a4eebe1dfa6a6caf8926e4e63a0d00a20a112adb5860ff3d057bd3b61365: Confidence: 100.00, Family: trojan
Some of the related sample hashes are:
14179a1c6bf196ddf9e0756edf53636cd90f7a0b4e54befbb0cd98afa111593e
05321e5c99fb81af6d2fb8ee61d31a7340b4b37000305fe56d81149588ce099c
3a5c9b8771095a5345f266789b88851fc5276f1d7292c0ff22398023cfa20b4f
bf0c0ae8dca0b191eb2b44736b11b8915ad928fddd0ed35ff11b53d0d42ff7ed
The Main entry point for both droppers is a BroadcastReceiver listening for the android.intent.action.BOOT_COMPLETE and android.net.conn.CONNECTIVITY_CHANGE events – these are “callback” notifications that cause the app to run when the device is restarted, or there is a change in network connectivity. When any of these events are triggered, the sample will start a service whose first action is to load a native library.
The native library registers a couple of native methods implementing the dropping, decryption and dynamic load of the payload contained in the assets folder. Interestingly, the analyzed droppers tend to use over-complicated approaches to obtain particular system information or performing common tasks.
For instance, both samples read /proc/pid/maps to check if any 64 bit libraries are loaded and find out it is running in a 64-bit capable system or use ZipFile and ZipEntry classes to load native libraries using the System.load(String path) instead of just issuing a simpler call to System.LoadLibrary(String libname) despite the fact that they are in the proper lib directory.
Once the payload is loaded, an instance of one of the classes contained in the dropped file is natively created using the reflection API. The instantiated class implements the Handler.Callback interface, which allows it to handle Message objects containing parcelable objects. The natively created object is returned to the dropper, which transfers the control to the payload by calling the handleMessage function of the object and passing a Message object with a specific code and a hashmap with some information about the dropper. The dropped payload is not too different from other trojans/backdoors in the wild, and includes some of the common behavior:
- Capabilities to install/update new applications and load other components in runtime; this feature includes the complete logic to handle installation errors.
- Checks for toolsets such as toolbox or busybox (indicated a rooted device) and attempts to abuse them to remount the root filesystem and modify system files.
- The sample uses several mechanisms to achieve persistency: periodic checks of the process list to check if the dropper service is running, deployment of a native component that silently launches the dropper service using the pm and am commands.
After our own analysis, we checked what other vendors know about the dropped payload. It seems it is labeled as a known trojan but there is no consistency among vendor labels. While some vendors claim this belongs to the Triada family, others label it as a generic backdoor. What it is definitely true is that it contains advanced capabilities commonly found in aggressive trojans and it poses a real risk to infected users.
Why are these samples still in the wild?
The success of a threat actor is often to cast widely and quickly. In order to do that, threat actors reuse old malware code to deploy new malware campaigns by modifying malicious features and applying different kinds of obfuscation layers and techniques. Obtaining the source code is not difficult. Several families, including Zeus, Bankbot, Maza-in have been leaked and are available in underground forums, accelerating the proliferation of lookalike malware (e.g. Anubis) that are rebranded versions of the original sample(s).
Threat actors prey on easy and vulnerable targets to distribute their malware. A common and effective way to distribute their malware is by targeting users and devices that are not protected by the app vetting processes offered by official app stores. Instead, threat actors rely on third-party app stores, enticing “free” and cracked games and apps, and phishing campaigns to distribute their malware.
Combining the ease of access to malware source code and the ability to modify its features, including the various distribution channels to seed their campaigns, it’s clear how threat actors are bypassing outdated AV signatures of “known” malware.
Staying ahead of the problem
We’ve proven through third-party testing and from the adoption of our engine by industry leading organizations (stay tuned for announcements on both the testing and industry endorsements) that Zimperium is a key threat intelligence source. And we are the solution for providing on-device detection and classification of zero-day malware. The ability to use behaviors coming from known sources to find similar features in unknown sources, and to cluster the malicious samples in malware families, are important traits to its success.
We constantly train our models with new data to be able to detect unknown, and also common and well-known malware families. Being able to quickly identify the most malicious samples in a bigger set of applications and divide them by family is fundamental for a proactive research environment and effective remediation policies.
As this research showed, we constantly correlate older unclassified samples with the latest threats to be able to spot similarities and eventually identify the early stages of known malicious campaigns that evolved during the time. This proved true for several of the samples, and we can speculate that some of the early stages were released by malicious actors to measure the efficiency of the spreading vector mechanisms and the AV detection coverage. Before Zimperium, the actors must have been pleased with the results.
Conclusion
In this blogpost, we showed again the outstanding detection capabilities of our machine learning-based detection engine, z9. This case also illustrates how z9 is highly resistant to common flaws of machine learning.
For instance, several machine learning approaches used in malware detection and other domains whose target changes along time, may suffer from concept drift, the difference between the statistical properties of the concept the classifier is trained to predict and the actual properties of the data it uses to make a decision.
This translates into a loss of accuracy as time passes. Periodic retraining using fresh data and a statistical analysis of the feature set are proper countermeasures to prevent these kind of issues.
The findings presented in our article prove our machine learning-based solution is resilient to these kinds of problems, and it is capable of making accurate decisions on both cutting edge malware campaigns and N-day threats.
We will be conducting a webinar on November 20th at 10am Central, where will be providing additional context.
IOC
SMS fraud (Avito)[1]
988c8608f4534694c515aa7b7f3ede21d3edf1bab6e095e1b5a088219502c7b3
7fb90d67ce900f99bf8cfcb4f58fe4bae1f56a08aa8681a41def3117b750b813
94d8fcc50fc4381d6a5634dffa019845c34af3de50eb757c6b97366007a22ae7
3d4cb0a0bbe3416f128560503704f73a7b2acb3a561c8636b5f38f877f1b8b45
3317fae9ed06922a5508689237f44fe3dfb56150b6f8c015fba5deb8525b5fab
c3c8d924849e2ad024de13314500864150f1343f5abe13d35633b7dcfad42029
2327b25dab0df2b8c75b59bc1947874b0888156d216d34c0531b827b52542958
4b48e4268b0befdfa3ce11c258d2fdd54096256312b3e31cc217994c30b8d123
2e2cac6f6e55d1eab273b73cf5b3b787d26b26eff101b6ee2fd9ab2ebb470d91
9cdf88883441bf5ff9e7c1f81326037d3f7d714444a9ce6dfd655db525a476ac
65f33e7ce09342f2eb22177619661f00d8b52ba4a5ad9f6a660d106ed6d76669
9909d3fc626bfe2c715a689d3a3e81a63ee5b892b2072042a53ed368d85e9cb1
0c4ec6b4a8e9674bf7af120b767477fdc460753eda860a73b270d08ff9b0c841
34c5cb6da391f1f14ec6760587b5d287ec5bff0fa42cd18b56825cf16d0a6cbb
0b4b9b25180b18f973d58b49bd6c8a2ef94d19ab0a884e6dfcac525b161e0c14
7a1cea64c6b413853864d8173bdc202b6602885c23e0cb727fdc4a737e68facf
3427862a88b3feb9a64bbfff087e519bcd9eca87f04fb4f70f715b12080c71b5
1102799da8429d6d6b63b8928c1de3b53219093003d256256d7766b1761ad7ed
9a34042d24244c4b9afb4cb1d98f6848ea8eb845c401d791b2fc15f514877363
Banker trojan (Bankbot/Anubis variant)[3]
ca0dad8c0d7daecd12de79f6ebabc33979e4c3dee688418b56efc01fe9b65bfc
579fe1f6a9991a9257fe0ca27692bf84286eeb4a9ba26c73ef9e8841390ca5d5
feaf2ccf3f228deada42cb4261ded94e5f92f91a76e2fc4e61b1f094a330da6c
7c2edd827709df4a5fef45c0b6556f0ade1ebfb120869e20ada7e707509a2df6
3e1037a2f12a32d7fafe8df4bf4e7837d7c001bfc166052f830ef3cd9518644a
de5b89309772848dbd78ac15c8bef71a2e8f8e7d9b843e173ee68de70afd1fd8
89f6e2ecfbd60f4edbdcb457313efd824379a9ea316a078995ac1eaf35fef270
Banker trojan (Bankbot/Anubis variant)[4]
26ace4765f2837a67c9158aa840698338593a35f217b5ea1f617153d4c98179f
5fc9deef21bcf80145ac0cb46b5c15479a39eece440f4abaaba59342029cb8bb
2a4c2c2e67408d0fef4dcc0c7a5a6dcb2cf1c1d3caa10f96b71572a5eee89a30
b5135a8a5d76297e505db9c6a02fb2262a79f9460f18f30ae93c2e6577f29139
Banker trojan (HQWar dropping BankBot)[5][6]
8322d5c6766fe87375afb32f48539bdf234c1adaacf889434dbaf059e2823095
Riskware/SMSPay/Dropper
f4200d09ec51259bbf26e9bed1cc96548fa3b0fb336f683cdf9e7219ff6fabde
30608d7dbc7d620c885225995b431a5d87e1948df99bb8687410071473a6322a
d1a968186faac22d756b217f1bba8fac307c47b54db000c4b50a4e40abbb952a
6d3d7a04374aeb2f8ac4bb109c2703dcf7e2511c02fb922b176f4a526428f4d8
0101cbc6d0b44a5ab638da7a8fbd7205e7b9f88c8b5d7b124d791a7bf389920e
2dabc2860f041fe551b434e5fb6d5de7c827408a1fd555ec1bb47c8eba762292
4453ede1cd5c22f9b55f740e3ebf305c346ca94b2a331aff550f4ef9f6b42813
b93fae996fc9d6ded5f201627ed09c736a98eba7db2dfb53c65b33f7bb759e63
6c76f15273d5f32f217b1a73003a41d55cf1fc95610633ff902aff9b873247dd
7697ed7549294c72464f4ff1f1739b3b52fa9dfe31760c8a959e21f3c7c05402
6985169f23a313e9526f9e6bad62025a9cb7fcee0cba87ab45409ea8a66c506d
c5fb49b5a250b63fa11b65e1b5708b0be8917736fe80aa37547810e6b494b972
f0ad4da325e610a1f448afff472cdaa9ca83ff51dc0ea57f129675e00e69d943
19c2534a23f3d3c0456ad6bfe576492f01c0f93d61c7c45ae635e2c126ac41a7
68d6c88fc804f17777db415fea7725cda70e743665da7ae0cda84bfcd34702a7
38fee213b8a1bbd407a7227028107e12c1114787f98769084fb6e2754aad1364
Ac1bd0dc8b45f9fc74fa471165ece2ccf8238444fde3750b54aa2c338f4e76f4